The new Ai-mbots.
- Andrew Hogan
- Mar 31
- 7 min read
Updated: 7 days ago
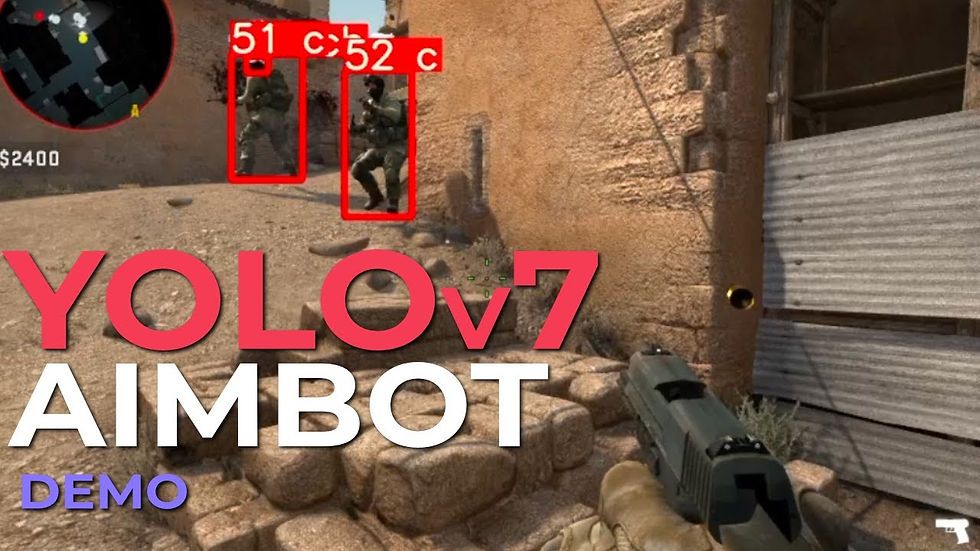
One outcome of continuing improvements to anti-cheat solutions, has been the increased interest in hardware and DMA cheats to get around detections and bans.
However, with these costing up to, and sometimes over $1,000 a go, they represent a significant investment - and there’s no guarantee they won’t be detected by the better kernel level anti-cheat software.
A more affordable way to get around the best efforts of publishers' security teams, and judging by our monitoring, one that seems to be growing in popularity, is the use of pixelbots - also known as colorbots and more recently as AI-aimbots.
The chart below shows the mentions of pixelbots across cheat communities in the PVP ecosystem monitored by Intorqa. Clearly there was an enormous spike over the 24/25 holiday period, but interest is continuing to grow year on year.
Which is why we're taking a closer look at them in this briefing.
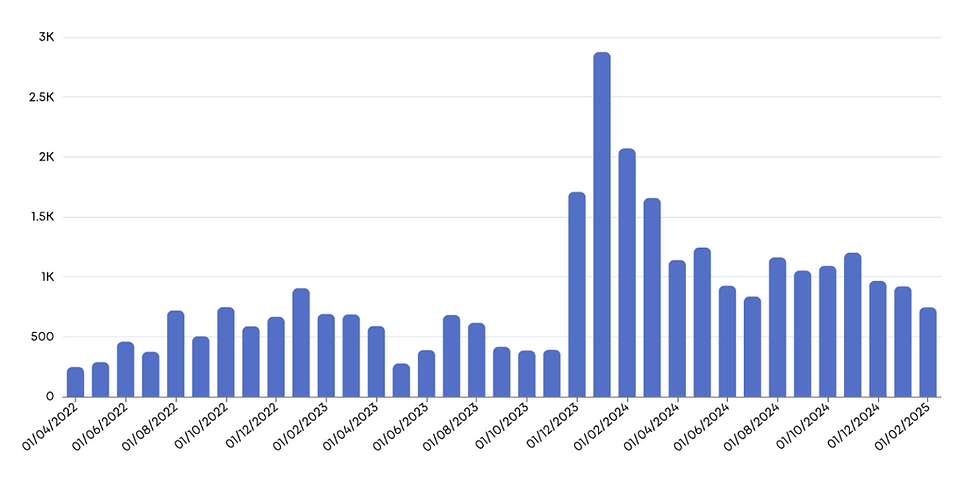
The first thing to say is it's not always been like this.
Even now pixelbots are often not taken seriously in the cheating and security communities, as they’re not considered to be very good or much of a threat.
When we ask prospective clients about what their priorities are, pixelbots often come pretty low. They’re seen as inferior to internal cheats, offering less functionality and features, and being slower - which is one reason they were traditionally more prevalent on MMO's vs FPS multiplayers.
But that's changing.
A quick reminder on how they work…
A pixelbot scans the screen so it can detect certain pixels that represent the target, and then aims by ‘moving’ the mouse to the desired position.
The name ‘pixelbot’ comes from when the very first versions would just look for a change of colour in a pixel on the screen, so they worked best when games had teams with bright colours e.g. a red and a blue team.
As games like CSGO got more sophisticated and realistic in terms of combatant uniforms, pixelbots worked less well. To keep up cheat developers would use learning models like Open CV in order to keep up with game developments, with the bots designed to aim for heads or similar targets, but as gameplay continued getting faster, they still struggled.
In their favour pixelbots don't rely on modifying gaming data while running; and with no DLL memory hacking or code injection, they tend to go undetected for longer due to not interacting with the game as much.
They also rarely offer rage features, so players accusing other players of using one are less frequent. Furthermore, unlike memory cheats, they will often keep working whenever a game updates; this adds to their appeal, as game updates generally knock working cheats over.
And then there's the price. As alluded to up front, another reason for the growing demand for pixelbots, is their relative affordability - particularly when compared to using DMA cheats or hardware like a Cronus Zen.
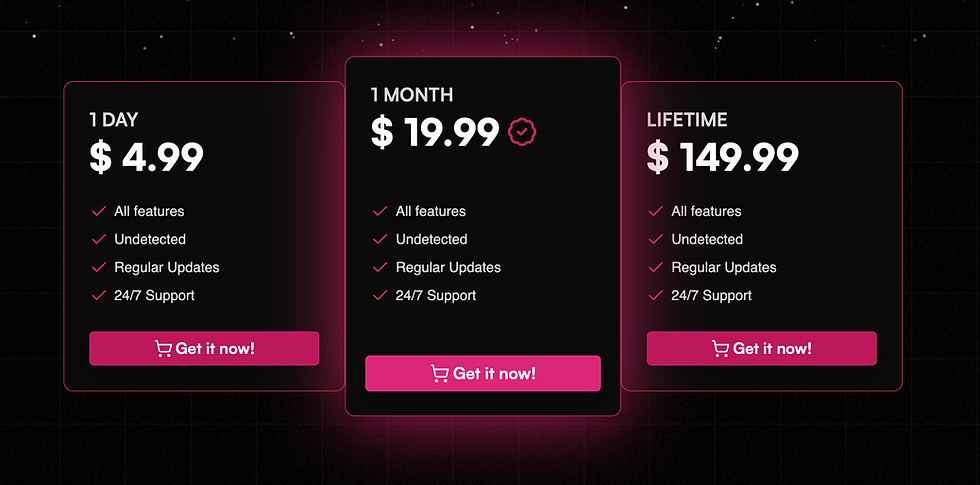

But the real game changer has been the continuing development of computer vision and machine learning over the last few years, specifically when it comes to object detection.
Object detection is a computer vision solution designed to identify the location of objects in an image or video with each identified object assigned a label that represents its contents. Using object detection, you cannot only spot the object in question but also count the number of times different objects appear in an image.
It was originally designed for more legit uses than developing cheats, such as helping guide autonomous vehicles, managing drug distribution in pharmacies, and counter terrorism. But that hasn’t stopped the ever entrepreneurial cheat devs from making the most of it, and one solution in particular - YOLO.
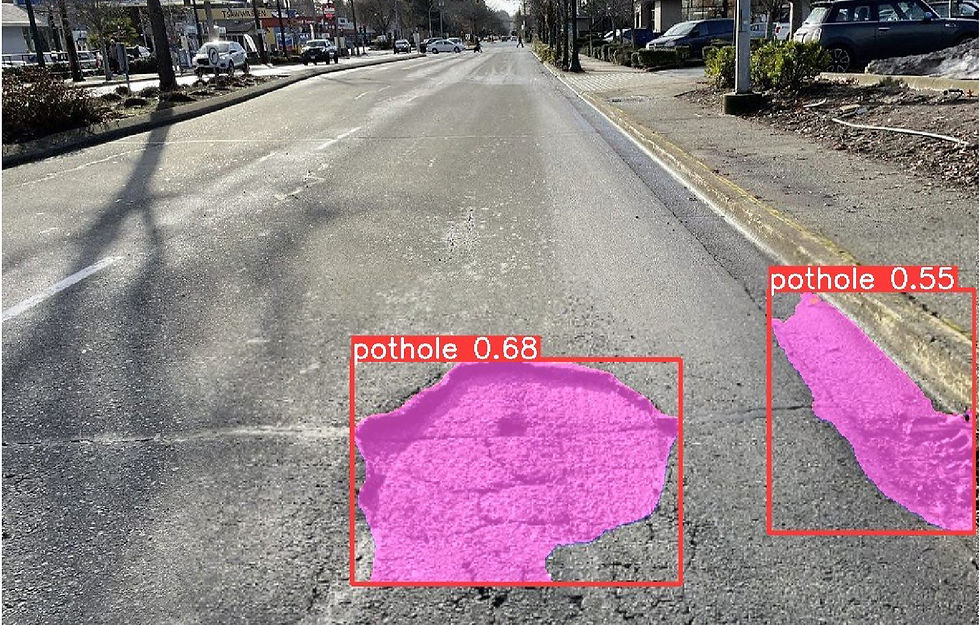

YOLO isn’t the only object detection solution, but it is probably the most popular one - and as a result the easiest to find. Of course, its promise of real-time object detection appeals to cheat developers, particularly with the latest iteration, YOLOv8.
In contrast to traditional methods that require multiple passes, YOLOv8 only needs a single pass through the neural network. This differs from two-stage detectors, which necessitate a separate proposal generation stage before object detection.
Single-stage detectors are generally faster and more efficient, making them perfect for real-time applications like aimbots in fast-paced shooter games. In these, AI-enhanced aimbots use computer vision and machine learning algorithms to analyze game frames in real time, identifying enemy players even in complex environments.
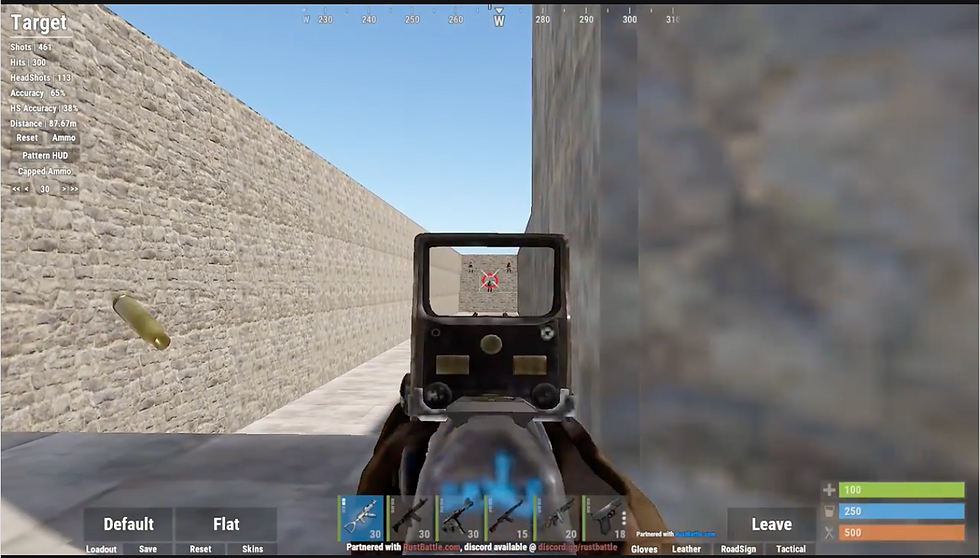
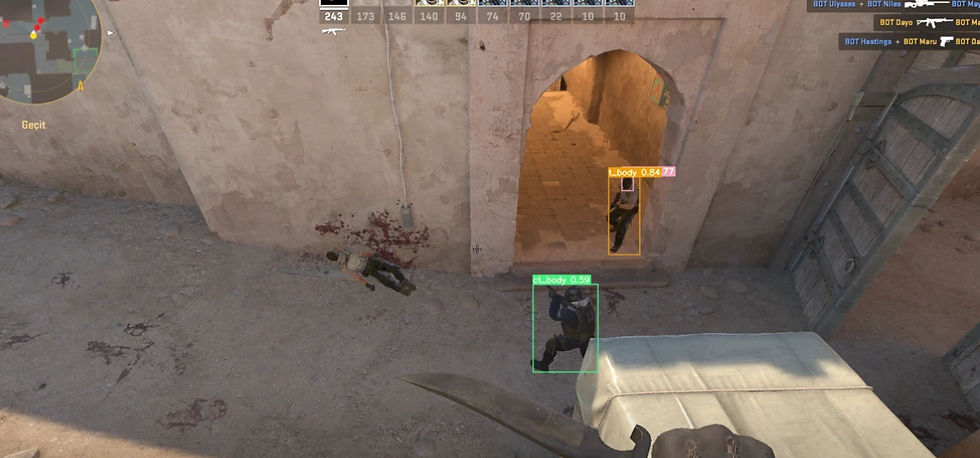
As with any object detection tool, training the AI model is a crucial step in the development of an AI-aimbot.
This involves supplying the model with labeled data, for example gameplay videos and images from YouTube or Twitch, which are annotated with bounding boxes around the objects of interest.
During the training process the model's parameters are iteratively fine-tuned by feeding labeled images to the neural network. The model's performance in recognizing various game elements improves with each training session and will eventually reach the required accuracy.
Even here advances in technology are making the developer’s job easier, as augmentation, pre-processing and annotation tools mean far fewer screenshots are required to develop a dataset, and a developer may not even need to do that. Sites such as Roboflow ('The world’s largest collection of open source computer vision datasets and API’s') offer pre-produced datasets and trained models.

One of the best known AI-aimbots over the last 12 months is AIMMY which is described by the developer as ‘a universal AI-Based Aim Alignment Mechanism… to make gaming more accessible for users who have difficulty aiming.’ rather than an actual aimbot - ie cheat.

It’s easy to be cynical about this. After all, using accessibility as an excuse for providing cheats isn’t new, but unlike the makers of hardware cheats like Cronus Zen, AIMMY’s developers do seem to be genuinely interested in helping gamers, who are otherwise ‘at a severe disadvantage over normal (their words) gamers’, such as those who are ‘physically’ or ‘mentally challenged’, or suffer from ‘untreatable visual impairments’.
That said, they also include gamers ‘trying to improve their reaction time’ and those who ‘perform poorly in FPS games’, which seems to broaden it out a bit too much, and would certainly include the writer of this briefing!
But as they say, it is free to use and not done for profit.
"This means no ads, no key system, and no paywalled features. Aimmy is not, and will never be for sale for the end user, and is considered a source-available product, not open source as we actively discourage other developers from making commercial forks of Aimmy. Please do not confuse Aimmy as an open-source project, we are not one".
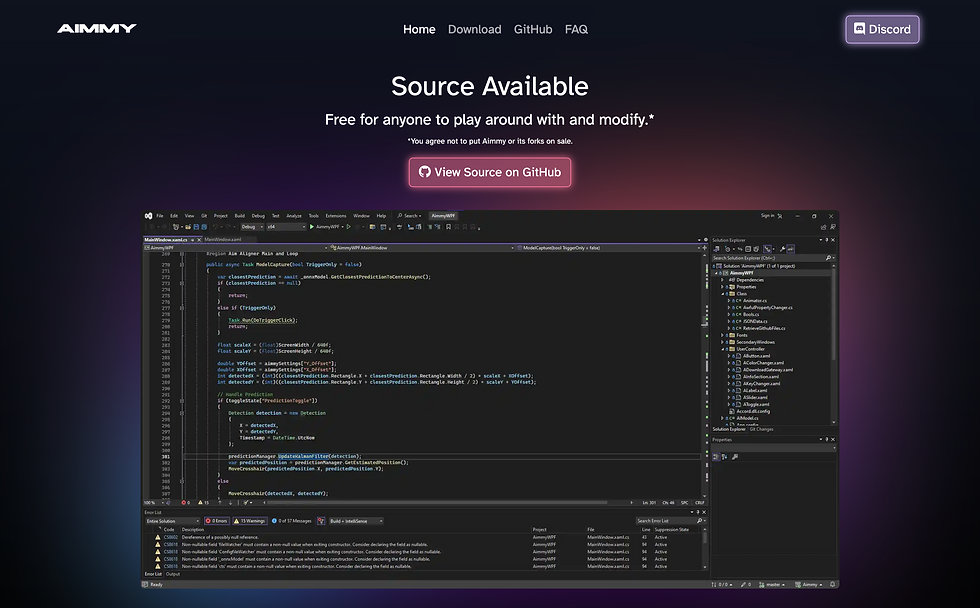
Unfortunately, AIMMY has proven to be very popular amongst cheat communities and is often discussed, shared and reviewed in various cheat forums, with many users doing exactly what AIMMY were trying to avoid when they decided against being open source.
Computer vision based cheats are continuing to get more sophisticated in other ways. In this briefing we’ve focused on AI-aimbots, but computer vision is already being used to create wallhacks and ESP, and not just on PC’s but consoles as well, where they challenge the likes of Cronus Zen.
These developments and the growing popularity beg the question: can these new aimbots be detected?
This is a familiar debate in the cheat communities themselves, as this question in Unknown Cheats shows:
‘Hi, I'm not using a pixel bot or any sort of external program. I am purely just curious on how an anti-cheat would be able to detect them. I was researching that anti-cheats look for "bot" like behavior in a player's movement and or inputs, but I want to know how they can even detect or find something like this if it's not messing with the game.’
Of course developers always claim their cheats are undetected and won’t get you banned. And to be fair, the logic that pixelbots will go undetected for longer, due to not interacting with the game as much, does make sense.
But crucially for video game publishers and their security teams, they can be detected, and ironically, AI is playing a part in this. And cheaters are finding this out:
‘The mouse input can be detected. Or it gets detected by AI. Let's say you always shoot 50 ms after the enemy is in your crosshair. Is that not weird? Tbh you don't even need AI to detect it.’
It’s about focusing on behaviour. Do this and you can find cheats regardless of the techniques.
Behaviour based cheat detection that analyzes player interactions and movements isn’t new, but as this quote implies, AI is making it more effective by enabling it to perform real-time analysis of a much bigger volume of player data, and identify patterns that are outside the bounds of normal gameplay with more confidence. Machine learning models track changes in a player's mouse movements, reaction times and accuracy, and flag deviations or patterns that are beyond human capabilities and therefore deduce a pixelbot is being used.
So detecting them is possible, and AI is making that easier. However, at the same time AI is also making it quicker and cheaper to make the cheats, and as the number of vendors increase, the cheats become easier to find and use.
So, how do publishers get ahead of the problem? Just as with developing effective cheats, the key is training - lots of training.
There’s a parallel in the LLM boom and how LLM’s are now being used to identify content that is plagiaristic - in effect becoming anti-LLM-LLM’s.
To do this they need to be continually trained on huge amounts of literature.
In the same way, video game publishers and their security teams need to continually train their anti-cheat on the latest cheats to ensure they can detect them as efficiently as possible.
To do this they need to build and keep up-to-date, as big a corpus of cheats as possible; testing them, understanding how they work, and what they are doing, and use this to train their anti-cheat to identify the bot behaviour.
And above all, treat pixelbots as seriously as other cheats.
Comments